PhD Defense
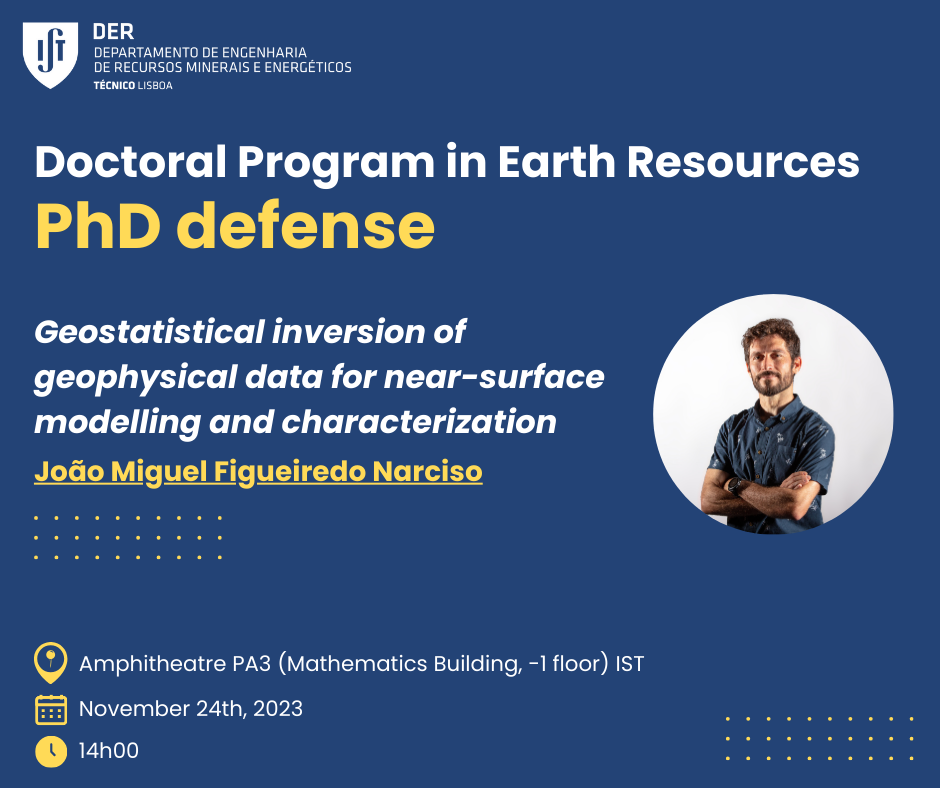
Candidate: João Miguel Figueiredo Narciso
Thesis Title: "Geostatistical inversion of geophysical data for nearsurface modelling and characterization"
Date: November 24th, 2023
Time: 14:00h
Location: Amphitheatre PA3 (Mathematics Building, -1 floor)
Research Supervisor: Prof. Leonardo Azevedo
Co-Supervisor: Prof.ª Ellen Van De Vijver
Jury
President: Doctor Maria João Correia Colunas Pereira
Jury members:
Doctor Thomas Hermans, Professor, Universidade de Ghent, Faculty of Sciences, Department of Geology
Doctor Amílcar Soares, Full Professor , Instituto Superior Técnico, DER
Doctor Maria João Pereira, Full Professor, Instituto Superior Técnico, DER
Doctor Giulio Vignoli, Associate Professor, Università degli Studi di Cagliari, Dipartimento di Ingegneria Civile, Ambientale e Architettura
Doctor Ellen Van De Vijver, Professor, Universidade de Ghent, Faculty of Bioscience Engineering, Department of Environment
Abstract:
The near surface beneath our feet is the portion of the Earth that affects and is more impacted
by human activities and yields important mineral and energy resources. It is, therefore, of the
utmost interest to characterize the first meters of the subsurface and to accurately quantify its
physical, structural, chemical, and biological properties. To overcome the limitations of direct
measurements obtained from invasive methods, non-invasive geophysical methods have been
applied in the modelling and characterization of complex and heterogeneous near-subsurface
environments. Particularly frequency-domain electromagnetic (FDEM) induction methods
have become one of the most widely used geophysical methods in near-surface applications
due to their versatility, cost-effectiveness, and data sensitivity to subsurface changes of two
physical properties: electrical conductivity (EC) and magnetic susceptibility (MS).
However, mapping geophysical data into numerical subsurface models concerns solving an
ill-posed and nonlinear geophysical inverse problem with multiple solutions. While
deterministic geophysical inverse solutions allow predicting smooth representations of the
subsurface, they do not account for uncertainties and are unable to directly integrate direct
observations, a probabilistic framework allows overcoming these limitations.
This thesis combines the advantages of FDEM induction measurements with the potential of
probabilistic inversion and introduces a geostatistical FDEM inversion method to
simultaneously model the spatial distribution of the subsurface EC and MS and assess the
uncertainty of the predicted results. The proposed method is benchmarked with an alternative
statistical-based FDEM inversion method. Since probabilistic inversion methods are
computationally demanding when solving for large-scale three-dimensional inverse problems,
the iterative geostatistical FDEM inversion is coupled with random tensor decomposition to
alleviate the computational burden. From a multi-geophysical inversion approach, this thesis
also presents a joint inversion method of electrical and electromagnetic data to reduce the
uncertainty of the predicted subsurface models in near-surface applications. The methods are
illustrated in realistic synthetic and real applications.
KEYWORDS: Near-surface modelling, Geostatistical FDEM inversion, FDEM data, Electrical
data, Joint geophysical inversion.